No Data, No AI: Bridging the Gap in Smart Manufacturing
Watch Webinar
Chapters
Webinar Overview
The buzz surrounding artificial intelligence (AI) and its transformative potential has reached a crescendo. While AI has already made significant strides in areas like healthcare, finance, and autonomous vehicles, manufacturers are facing unique challenges. Unlike some other industries, they grapple with bringing disparate data sources together, ensuring interoperability, and integrating edge data into cloud and enterprise systems for AI utilization.
Despite the allure of AI as a magic wand for solving data quality and trust issues, the reality is far more nuanced. In this webinar, Ravi Subramanyan, HiveMQ Director of Industry Solutions Manufacturing, will shed light on:
The importance of having a framework in place for reliable data
The critical role of data management and quality in feeding AI/ML and other advanced analytics models
How organizations can leverage AI as an enabler
Watch the webinar to observe discussion around how a strong data management foundation is essential for AI success, particularly in the context of Manufacturing and Industrial Automation. Don't miss this opportunity to gain insights into optimizing your data infrastructure for AI-driven innovation.
Key Takeaways
AI emulates human cognitive abilities using algorithms, large datasets, and computational power and is expected to contribute $15.7 trillion to the global economy by 2030.
Key use cases for AI in industrial applications include predictive maintenance, optimizing operations (OEE, supply chain, product design, etc.), anomaly detection, demand forecasting, and visual inspection.
Data is the lifeblood of AI since AI requires huge, high-quality datasets that are normalized and contextualized to perform effectively.
The data management market driven by AI needs is expected to grow to $513 billion by 2030, spanning data ingestion, storage, transformation, analytics, governance, security, and orchestration.
Traditional industrial data integration relied on brittle point-to-point integrations between systems like sensors/PLCs, SCADA, MES, ERP, cloud apps, requiring costly custom coding.
MQTT is the de facto IoT messaging standard protocol, enabling efficient publish/subscribe data movement, originally created for remote monitoring in oil & gas.
The HiveMQ Platform provides an enterprise MQTT broker along with components like Edge to translate protocols and bring data into MQTT, and Data Hub for data normalization/transformation.
The Unified Namespace (UNS) concept provides a hierarchical semantic model to create a contextualized single source of truth for all manufacturing data sources across a company.
An MQTT broker is a natural fit for implementing a UNS by creating topic hierarchies mapped to the industrial operational hierarchy (site > area > line > cell etc).
The live demo showed data flowing from machines through Edge protocol translation and Data Hub transformation before sending to a factory broker for integration with databases, streams, AI models, etc.
The demo also showed an AI bot querying the UNS topic hierarchy to extract operational insights like average humidity across silos, illustrating the value of contextualized data for AI.
HiveMQ provides security through encryption, access control, and integration with existing enterprise identity and policy management systems.
Transcript
Introduction
Erin Musselman: 00:00:04.850 All right, it's time to get things started. Hello, my name is Erin Musselman, and I'm on the Marketing team here at HiveMQ. Welcome. Good morning, good afternoon, and good evening to everyone joining our webinar: No Data, No AI, Bridging the Gap in Smart Manufacturing. Today, our speakers, Ravi and Magnus, will shed insights into optimizing your data infrastructure for AI-driven innovation. You'll see a demo showing data flowing from edge to cloud as a glimpse of what this could look like in a smart manufacturing use case. At the bottom of your screen, you should be able to see your Zoom toolbar. There you can find your Zoom controls, such as the chat, Q&A, and other tools. If you have any questions during the session for the speakers, please submit them using Q&A. For discussion, please use the chat. But if you're having any problems, please send me a direct message. We will be recording this session and sharing it with all of you following the webinar. And now without further ado, I'll pass things over to the speakers, starting with Ravi so they can introduce themselves and kick things off. Thanks again for joining, and I hope you enjoy the session.
Ravi Subramanyan: 00:01:07.864 Thank you. Thank you, Erin. And welcome, everyone. Hope you can see my screen here. Erin, if you can give me a quick thumbs-up?
Erin Musselman: 00:01:14.087 Yeah, looking good.
Ravi Subramanyan: 00:02:04.378 Thank you. All right, so welcome everyone to this webinar around No Data, No AI. And I know it's a little interesting title, but at the end of the day, that's what it is, right? So we're going to be taking you through some of those aspects of data management and what we need to do to ensure that you're ready for AI. So first, let me introduce myself. I'm Ravi Subramanyan. I'm the Director of Industry Solutions for manufacturing and some of the other key verticals that HiveMQ focuses on. And we'll talk about those verticals and what HiveMQ is and how do we kind of help with the data management and things like that. But I actually have worked closely with our salespeople as well as with the end customers and prospects explaining what they need to do to be prepared for this and how HiveMQ can help better serve them, so that's me. And then Magnus — if you don't mind introducing yourself?
Magnus McCune: 00:02:07.683 Thanks, Ravi. Absolutely. My name is Magnus McCune. I'm a senior IoT Solutions Architect on the Professional Services Team here at HiveMQ. Excited to be here with you today and look forward to this presentation.
Ravi Subramanyan: 00:02:19.326 All right. All right. And Magnus is going to be giving us a wonderful demo of all of the things that I'm going to be talking about, so that's exciting. So with that, let's just jump in here. So let's kind of define what AI is, right? I know there's multiple definitions. To me, AI is basically a field of computer science which has technology that basically emulates what human beings can do. Right? It uses the power of algorithms. It uses the power of the large data sets and the computational power of computers to be able to process things and do things that typically human beings are able to do well, if you will, through their cognitive skills or their neural networks and things like that — that's what AI is. And AI is actually getting very, very popular. As you can see here, PwC has predicted that there's going to be a staggering 15.7 trillion contribution that AI will make to the global economy by 2030, that's huge, right? And that's why everybody is talking about AI and how that can help them to get to the next level, whether it's industrial companies or whether it's consumer applications and things like that, right? So you have different aspects of avatars, as we call it, of AI, right? So right from processing chatbots to LLMs, state-of-the-art, which is basically large language models like the ChatGPT and Google Gemini and others, state-of-the-art diagnostic tools that can help you predict failures as it happens, or maybe it can do — count some numbers and tell you the probability of something happening or not if you will. AI is well and truly entrenched in the texture and feel of the world around us.
Key Use Cases for AI
Ravi Subramanyan: 00:03:59.483 As great as AI is, it's only as good as the data that is fed to it. And that's because, again, the AI needs a huge data set to be able to do what it's doing, right, and that means that the data should not only be high quality or huge data set, but also the data needs to be normalized, which means that every data set coming together needs to kind of look the same, feel the same. It has to be contextualized. What is the context of the data, right? Where is it coming from? And what is the latest value? What is the history of the data? AI is able to perform its tasks efficiently. So looking at the use cases, we briefly talked about it, right, there's multiple, multiple use cases of AI. I'm just showing a cross-section of the use cases of AI in industrial applications that we typically see. For example, in the connected car space, it helps by helping predicting engine failures or optimizing the battery performance or providing diagnostic information and using that to predict when potentially the car might have some issues around certain things, right, so that is in the connected car space. In manufacturing, which is huge, as you know, it's all about ensuring that you are able to have high overall equipment efficiency and by predicting machine failures and ensuring that you're able to do some maintenance before those failures happen. For example, helping you assemble products more efficiently by using robots and cobots, helping streamline your supply chain and helping you design better products, if you will, on the fly, to be able to change things, depending on customer demands, using data that's coming through different systems.
Data is the Lifeblood of AI
Ravi Subramanyan: 00:05:48.958 In oil and gas, it could be reservoir analysis or drilling optimization using the power of robots and drones to be able to monitor things and use all that data to figure out what are the right ways to optimize your oil production, reducing emissions, being more safe. In logistics, it's demand forecasting. For example, damage detection on products that are coming in, enabling digital visual inspections, automating warehouses, and many, many, many other use cases. I can be talking at length just on the use cases, but we got to move on here. So let's talk about data. And we briefly talked about why data is the lifeblood of AI. And it is absolutely important. In the next slide, I'll actually show some numbers from IoT Analytics on the data management aspect and how that has been hugely influenced by the influence of AI. So again, if you look at AI and data science, it has various things that you need to do, right, you need to collect the data, you need to move the data, you need to transform, label it so that you can do some anomaly detection and then learn and optimize, and that's kind of a cyclical process, if you will, right, and every step of the way, data is needed, and large sets of data is needed. Large sets of good data is needed so that your machine learning models, for example, or any continuous learning model or predictive algorithms or descriptive algorithms can do its thing. And so at the end of the day, it's about diverse data, comprehensive data that can be used to be able to fuel the AI. That's what it is, and of course, we'll talk about different aspects of how we can ensure that the data is properly managed to allow this AI to be effective.
AI Driving Data Management Market
Ravi Subramanyan: 00:07:49.291 So this is a chart that just came out. It's hot off the press from IoT Analytics. And thanks to our friends from IoT Analytics for putting this out. So it's basically talking about the data management market, right? So the different aspects of data management, and these are different applications that do data management, and how that market is expected to grow to $513 billion by 2030. And it's purely driven by AI and then the growth in AI and the importance of AI, right? So again, AI relies on different aspects of data management, including the different data sources that have data that's needed for AI to do its thing. Ingestion of data, storage of data, transformation, analytics, governance, and security, which is absolutely important, and orchestration. So all of these things need to come together for your AI to be effective. So let's look at the case of manufacturing specifically, right? So typical manufacturing systems follow what we call the ISA-95 model, where right at the bottom, you have your sensors and actuators that are on the machines that are collecting all of the data, if you will. Then you have the Programmable Logic Controllers that take all of this information and it performs certain logic. And then you have the SCADA system, supervisory control, and distributed access systems that are able to talk to different subsystems within your factory. And it's able to do some analysis if you will. It typically is paired with a human-machine interface, HMI. And then as you go beyond that, you're getting away from the realm of what we call traditional operating technology system into IT systems, which is now MES, Manufacturing Execution Systems, and ERP systems. So this is kind of the typical value chain of information that is stored in different levels.
Traditional Industrial Data Integration
Ravi Subramanyan: 00:09:45.102 And unfortunately, in a traditional industrial data integration system, the data is siloed, right? So your shop floor has the information specific to the machines. The SCADA layer has some information that is covering certain subsystems. MES has information regarding the execution of the manufacturing process and how to do that. ERP has information about the order information about parts, for example. And then you have the cloud that has more enterprise-grade information that is running dashboards. So to be able to send information from the shop floor to top floor, as we call it, you need a lot of point-to-point integrations, so each level has to be integrated to the next level. So you cannot go directly from, say, the shop floor to the cloud, right? And that would be ideal, but you cannot do that. So this is where I think a lot of custom development by system integrators came into play where they would actually develop these integrations between the shop floor and SCADA, SCADA and MES, MES and ERP, ERP, cloud. You get the idea, right? And so that they ultimately can — the data can find its way to the cloud. And guess what? These integrations are, again, very proprietary integrations. The moment you go to a different subsystem within your factory or other factories, you have to restart these integrations. You have to start from scratch, which means that a lot of these efforts that you put in on money and cost, if you will, are a throwaway effort. So that's not ideal. So you want to be able to have a system where you can reuse some of the data. Each of these systems are talking to each other in a succinct way, and you can reuse a lot of this data integration. And factories are not just like, okay, you set up the factory and you're done. New subsystems keep coming, new points of data sources keep coming.
Ravi Subramanyan: 00:11:35.152 So you want your system to be able to easily integrate new data sources, new subsystems, new machines that come in, for example, and not have to keep doing these data integrations to all of the data to flow. And so that's kind of what we show here. A traditional data integration is like if you want devices to talk to applications, PLCs to talk to analytics applications, gateways to talk to MES systems, typically, you do like point-to-point communication, which means that the device has a direct connection, like what we call a client-server model where one of it is a client, the other is a server. The server is like purporting the client for information, the client is sending the information, and then the server takes the data and does stuff with that, so you need this point-to-point integration. From a bandwidth perspective, maybe if you have limited connections, it's okay. But as you keep increasing the number of connections, which as you know in the case of a factory — it's very, very complex — it can get into a very, very spaghetti-like architecture, so it's not efficient from a bandwidth perspective. Imagine if I need information just say once a day, the analytics information needs information once a day from the device. What's the point in me creating a point-to-point connection and keep pulling for that information all the time, hogging the bandwidth just for that one piece of information that you need maybe once a day or twice a day, right? You rather kind of like get that information when it's available so that you can do stuff. So that's kind of like where traditional architectures and data integrations don't quite work between the OT and IT systems. This is where MQTT comes into play, right?
MQTT — the De Facto IoT Standard Protocol
Ravi Subramanyan: 00:13:12.822 So MQTT is like a de facto IoT standard. It started in the late '90s. It was actually started by IBM for an oil and gas use case with Phillips 66, where they were dealing with remote assets, upstream oil and gas assets like oil wells in the middle of the ocean or in the middle of a desert, where connectivity was very, very choppy, bandwidth was limited, but they wanted to bring the data to their enterprise so that they could do remote monitoring, for example, for two main reasons. One is like from a safety perspective — didn't want to keep having to send a person in any time they had to fix things because A, it was costly, B, it was unsafe. And they also wanted to kind of have a tab on all of their remote operations in one location. So that's why MQTT was actually created as what we call a publish/subscribe-based technology, as opposed to the client-server model that you saw there, where if I'm a publisher and I have some information to publish, I'm a client and I'm publishing that data to the broker, the broker takes in that information, and then it shares that information with other subscribers that need that information. And typically, the publishing happens on a particular topic. For example, if I'm a vehicle and I'm trying to say my temperature or the pressure values on my subsystem is so much, somebody or some client needs to get that information. Maybe like a mobile application needs to get that information or a backend application server needs to get that information to do what it needs to do. So it's basically published on that particular topic, and then the subscribers that are subscribed to the topic automatically get that information as they publish-subscribe. So I don't have to talk to the publisher directly and hope to get that information. I can automatically get it.
The HiveMQ MQTT Platform
Ravi Subramanyan: 00:15:03.785 So it's a very efficient use of my bandwidth. It's an efficient use of my resources. And very quickly, from oil and gas, it moved over to many, many other industrial applications and even consumer applications. For example, Facebook Messenger is using MQTT as well to manage all of the messages that flow through. So because it's highly scalable, it's highly reliable. It is very efficient. It actually now is an open source, as of 2010, it became an open source. So anybody can develop to the MQTT standard, and all publishers and all subscribers in the ecosystem can easily talk to each other because it's all — as long as you adhere to the standard. And the standard is getting very, very popular as a messaging protocol because it's lightweight, it's efficient, as mentioned. The packet sizes, the message sizes are very, very small. It's only 200 MB, which is really small, and it's bidirectional. So publishers can be subscribers, subscribers can be publishers, and it is actually designed for stateful content, right? So where you can actually retain the state of where you are, right? I mean, am I still there or am I gone? What it is, right? So it's very aware of that. And so that's MQTT. And so this is the HiveMQ Platform. HiveMQ is predominantly an enterprise-grade MQTT broker solution. And it was started in 2012 right after MQTT became open source. And the biggest thing that we differentiate ourselves is our high scalability, high reliability, and high availability. And we are able to support multiple use cases. We'll talk about the industry verticals that we are in and also the large portfolio of products that we provide. Of course, our main bread and butter is our broker, which is right at the center.
HiveMQ Industry Verticals
Ravi Subramanyan: 00:16:54.717 But we also have now incorporated other solutions that help you really get the data from the sensor to cloud. For example, one of the products that we recently introduced is HiveMQ Edge, which was initially available as an open source — and it's still available as an open source — but we also added some additional features to commercial offerings on top of that. Basically, the idea there is that these different devices — be it a windmill, be it a robot or a factory machine or a sensor — they have different protocols that they talk in, right? Some of them may be kind of like the serial protocols like Modbus, for example. Others are digital protocols like Siemens S7 and Rockwell Ethernet IP protocol or OPC UA, which is pretty common from a machine-to-machine communication perspective. And so what the HiveMQ Edge does is that it can translate all of the data from the native format into MQTT. It can bring the data into the central broker. And imagine this Edge can be — there can be multiple Edges. For example, in a factory environment, each subsystem can have its own Edge that's consolidating the data, bringing all of the data into the central broker, which typically is in an enterprise location, or it could be on the cloud as you can see at the bottom. And then it can bridge that data into other systems like databases, streaming systems. It can add in additional security. You can apply your own security postures, if you will, on the broker. And you can also have custom applications or extensions developed based on the SDKs that we have. So that's kind of our biggest thing. The high scalability, high reliability. High availability, that's another big thing that we do as well. And so these are the different industry verticals that we focus on. So connected cars and mobility, that's a big one we started in that space.
Foundations of Unified Namespace
Ravi Subramanyan: 00:18:51.345 But we also have a huge footprint in manufacturing and industrial automation in various kinds of sub-industries like pharma manufacturing, auto manufacturing, semiconductors, you name it. Transportation and logistics, that's a big area for us. Wherever there is large amounts of data and there's high scalability needed, we are there. And the last but not least is energy, which is oil and gas, renewables, and others. Now let's talk about Unified Namespace and what role does it play in the whole data management aspect, right? So Unified Namespace is basically a framework that was coined by Walker Reynolds. The idea is that you have this common data infrastructure where you can bring all of the data that you have within your subsystem and can create a contextualized view of all of your data. Let's say you have your machine data coming in from your different machine subsystems. You have your alarms coming in from maybe an MES or some other system, CMMS or MES system, alarms, and alerts, for example, specifically for a factory. And then you have other pieces of information coming in from, say ERP, specifically, information around the parts and the quantity and the forecast and things like that that's coming from there. So for you to be able to run your business, you need a snapshot of all of this information and not just a single piece of information, right? So this kind of creates that common data infrastructure. It creates a contextualized view of all of the data where you can bring it all together.
The Core of Unified Namespace
Ravi Subramanyan: 00:20:25.677 Now, naturally, when you look at this diagram, you clearly see a parallel between this and MQTT that we saw. So an MQTT broker naturally lends itself to creating a Unified Namespace very easily because it is able to create a single source of truth where different kinds of information can come together and you can actually see a snapshot of everything in one location. So again, at the core, you have the broker itself, right? So the broker that is actually getting the data, you have some kind of a platform that is doing the data modeling, the contextualization, normalization. It can bring in different kinds of information. We talked about the plant flow data. You could have flat files. You could have the database files. You could have other information coming through RESTful APIs. And then ultimately, it's all about sharing that information with other systems, if you will, and with what we call the nodes, are bringing in information from those nodes and creating that single source of truth, that AI applications can easily use this information. So A, the quality is high, B, it's all ready for them to go, and it doesn't have to do all of this data manipulation to be able to run your AI models. And back to the diagram that we showed earlier, and now kind of juxtaposing that with the UNS, where now everything is all interconnected, right? So now, you don't have the data silos anymore. Everything is interconnected through our system. So HiveMQ obviously is a big MQTT broker and we offer Unified Namespace solutions where we can actually come in and offer different levels of Unified Namespace, bring all the data together, connect all of them through what we call our bridge, and then make sure that you have the data available at different levels, and you were able to provide access to the data at different levels based on what level of access you want to provide to the personnel — different kinds of personnel that need access to that data.
Reference Architecture Model and UNS Semantic Hierarchy
Ravi Subramanyan: 00:22:30.279 So, typically, the way Unified Namespace is organized is basically borrowing from the way ISA-95 model is set up where you have the enterprise. Then under the enterprise, you have the site, you have the area, the production line, a work cell. Literally, when you look at the sensor data, you can trace it back to where the sensor data is actually coming from. So you get the context around the data, and that's what Unified Namespace can provide. It's around the context. It's around ensuring that you have the latest values of the data that you can then trust and use it for your AI modeling. And this is kind of like a snapshot of a dairy farm and how they've done their Unified Namespace, right? So again, back to this diagram. So the enterprise, site, you have different packaging, for example, packaging division, and then underneath that, you have created a Unified Namespace called profitability that has different items that are needed to be able to gauge that. You have packaging and then you have scheduling. You have lines where you have different subsystems. So there are multiple different ways to bring together the Unified Namespace that is able to then drive different things and different use cases that you might have to be able to run your AI or you can use it for other use cases within your enterprise. And this is kind of like showing it in a slightly different view, as well, where the broker is able to kind of organize all of the data in a way that makes sense. So if OEE, for example, is important, so what are the pieces of information that you need for running your OEE? Maybe you need the temperature pressure and a few other information. So you can create a namespace for that and then that can be easily tracked. And all of the data sources that are needed to pull that data are automatically brought together to be able to help you run your analytics or run your whatever use cases that you have.
Where Does the Unified Namespace Live?
Ravi Subramanyan: 00:24:24.702 And this is kind of showing overall from a Unified Namespace perspective, data management perspective, from a manufacturing — so you have your end devices, sensors that are running different things. Then you typically have your gateway that is coming — what we call Edge of Networks nodes because your devices may or may not understand MQTT or the language of the Unified Namespace. So you typically have these translators that come in the middle, which are the gateways that can take that information and translate it into a format that is understood by a Unified Namespace. In other cases, it could come directly, like a PLC maybe that could be already enabled, that can bring in the data, or a device that could be enabled. You could build a central SCADA system if you will, that can use all of this information and run your SCADA from a central position. And then you have all of these applications that are using that information. And one of the things that I did not mention here is Sparkplug, which is a data framework that sits on top of MQTT that adds more information in terms of the way data is organized on top of MQTT. There are some limitations of Sparkplug, and when Magnus gives his demo, he'll talk about how to overcome some of those limitations. But what it basically is providing is, again, a framework for being able to share the context of the data and be able to see the way the data is organized. You can do state management, for example, whether a subsystem is born or dead and what state it is in — so that from a manufacturing perspective, it adds a lot more context behind the data as opposed to MQTT, which just sends you — there's no hard-and-fast way to create the topic namespace, the topic, and then the content. You can do it however you want. Whereas, in manufacturing, you need some rigor because there are specific ways the data is organized. And Sparkplug aims at providing that rigor to the customer as well. So that is Sparkplug.
Ravi Subramanyan: 00:26:30.994 And I believe that's all I had from a presentation perspective. Why don't I hand it over to Magnus? I'll stop sharing Magnus. If you want to take it over and do your demo, please go ahead.
Magnus McCune: 00:26:43.457 Thanks, Ravi. That's excellent. Wonderful presentation. I think you contextualize a lot of what I intend to share as part of the demo. So I think that's really fantastic. All right, folks. I'm going to go ahead and share my screen. Let's make sure I share the correct one and go ahead and do that. Wonderful. Can you see my screen? Can I get a thumbs-up from Ravi or —
Ravi Subramanyan: 00:27:04.356 [inaudible].
Demo: Enabling Relevant IIoT Data for AI Use Cases
Magnus McCune: 00:27:05.189 Excellent. Thank you, Ravi. Wonderful folks. So the demo that I intend to do today is sort of walking through what this might look like in a manufacturing scenario in a manufacturing use case, taking that data all the way from the edge, right where we're collecting and right where it's being generated within a device or at a PLC, wherever that data may live, all the way to our cloud systems, all the way through that data flow and the different points at which we can augment, normalize, rationalize data so that it's ready for an AI use case — where it's ready for some sort of process to perform further analysis or turn that data into information or even into insights that can then be used for value creation or value generation. The screen that I'm showing here now is our demo platform here at HiveMQ. So this isn't our actual product. This is a platform that I use for showing a demo and walking you through it during a webinar. Thank you, Anthony, if you're watching for building this, it's a wonderful platform for us. As I walk through this, I'll go into some of our actual products. So I will be opening up HiveMQ Edge and HiveMQ Enterprise and explaining why I'm doing so as I do so, but I just want to contextualize that. So I'll actually start off on the far left. And the title of this presentation is No Data, No AI. I think I'd further augment that and say No Data is bad. Bad data is worse. So not having the data means you can't generate insights. Having incorrect data means you generate incorrect insights, and so it's really important that we're able to fix data as close to the source as possible. And that's one of the things I'll demonstrate with Edge. As we continue with this flow, we then end up in a situation where we have a centralized location for an entire factory. So on the left here, we have my sort of factory site, so an individual location.
Magnus McCune: 00:28:57.611 And within that factory, I may also want to generate insights and derive things like predictive maintenance or something like image-based quality inspection. And so I might have some AI models or some systems running within this factory. And that's where this centralized broker within the factory lives. So all of my lines, all my individual tooling is flowing into maybe a centralized broker for that site. And from there, I'm able to further integrate additional systems. So when we look at this piece here, we'll be talking about how some of that might work and where that lives. And then lastly, generally speaking, we're going to want to flow some of that data through to a headquarters or a broader Unified Namespace for the whole enterprise. And that's really either our HiveMQ Cloud services or possibly a HiveMQ Enterprise broker running within a centralized data system. So I'll speak to each of those pieces, and what that looks like, and also where some of the AI integrations may live. Starting all the way off at the Edge, I'll open up our Edge software and sign in. Our Edge software really fundamentally does three things. It's looking to get data out of machines. It's looking to normalize, standardize, or in some way contextualize that data, and then it's looking to flow that data to a central system. That's really the goal of our Edge software. And I'll show how each of those pieces works together. The very first piece is our protocol adapters. The idea behind these protocol adapters is right there at the edge, right there on the machine floor. We have technologies that may or may not actually speak MQTT. They may not have the ability to communicate using the MQTT protocol. So we may have things like, as Ravi mentioned earlier, Siemens S7 or Rockwell, Alan Bradley ControlLogix, or CompactLogix devices, Modbus, OPC UA. So those protocols are not MQTT protocols, so we need some way to adapt them and bring that information into our centralized system.
HiveMQ Edge and HiveMQ Data Hub
Magnus McCune: 00:30:57.804 So our Edge software offers these protocol adapters. They're, of course, open source, and you can use these as part of a deployment. Ravi mentioned earlier, but Edge is an open-source technology for us. There are some commercial components and I'll speak to those when we get to them, but it's important to know that. So we have this data now flowing in. So for that first goal of getting data out of machines, we need that data in order to generate those insights. We've accomplished that using our protocol adapters, or if they speak MQTT, then we're directly connecting those into our broker. Our next step is that idea of data normalization. So fixing that data as close to the edge as possible. Once again, No Data is bad, bad data is worse. And so we have a tool set, and this is our first commercial piece of edge that I want to mention, which is our Data Hub component. And Data Hub exists in each of our products. So it exists in Edge as well as our core broker, but I'll demonstrate it here on Edge because I think it's a little bit more intuitive. And I have a draft policy here that I've built and that we can talk about very quickly. So in this case, I have a topic filter. So I'm looking to specifically trigger this data policy. So Data Hub is a policy engine. I'm looking to trigger this policy against this topic here, Global Industries, Munich, storage. And then I have a wildcard character, we can ignore that for now. And then parameters and temperatures. So I'm working with our temperature data in some specific way. I'm then running a data policy with a policy validator, and I'm validating a schema. So I'm saying, "Hey, let's make sure the data that is flowing through on this topic matches this schema." And in this case, I have a temperature schema defined here, and this temperature schema is actually quite simple. It's just indicating that a temperature value flowing across this should be formatted as JSON and it should look roughly like I have a value for that and I have a timestamp composed in there and both values are required.
Data Hub on Edge
Magnus McCune: 00:32:54.431 So the actual temperature value is required and that timestamp is required. So if, for whatever reason, the data that I'm flowing doesn't match that data policy — so on error, if it fails that data validation, I'm just going to drop that message. Once again, No Data, no AI, but bad data, worse. We really want to make sure we don't have that bad data. So anything that doesn't match that schema, we're going to drop. From there, we can also run transform operations against that. So we can say, "Hey, my timestamp is there and my temperature is there, but maybe that temperature is actually coming through in Fahrenheit and the rest of my systems want to use Celsius." So I might want to run a JavaScript function that is going to convert that temperature from Fahrenheit to Celsius so that all of our systems are reporting data in the same way. So I can run a quick Data Hub transform operation, and I just have a temperature conversion here where I'm doing the very simple Fahrenheit-to-Celsius calculation, and I'm converting that data to Celsius. So once again, right at the edge — before this data gets to the cloud, before we're trying to spend expensive hyper scaler compute on converting this data — we're fixing it right here at the edge, right where it's being generated. And this is a relatively simple conversion, but this could really be anything. Our Data Hub Engine uses JavaScript functions. If you can write it in JavaScript, you can run it as a function here and do that data conversion and normalization. And then just going ahead and redirecting that to a new topic that contains that valid data. So I'm still publishing it through to the rest of my data system, but I've now fixed that data. So once again, in order to get those good AI insights, we need good data. This is ensuring that for us. So that was the second function that I mentioned that Data Hub does. And our third function is flowing data into central systems. It's no good to me here at the edge. I need it within my centralized factory broker, and so this last piece is the HiveMQ Bridge that Ravi spoke to earlier and that's relatively straightforward.
MQTT Bridges
Magnus McCune: 00:34:53.781 We're just saying, "Hey, all data that is flowing through on any topic within this Edge instance, I also want to send that across to my broker." So I just want to send that across to the centralized broker and keep that bridge flowing data centrally. Once it's here in our central broker, this is where we might have more powerful AI models. So this is where we might be connecting in a system to do things like predictive maintenance or maybe image-based quality inspection or something along those lines. You might run those things here at the edge as well, that's entirely possible. This is a functional broker as well, so you could integrate those systems here, but this is where you might generally run more powerful models or more complex systems. We might also be integrating with additional data sources. So we might be integrating with something like a Postgres database, or as we can see when I open up this broker instance, I actually have a Kafka system here that is both taking data out of this system, so flowing data out, but I'm also passing this data — I'm also receiving data from Kafka. So I have Kafka to HiveMQ and HiveMQ to Kafka flows. So I might be processing additional event data, and then once again, an AI system might be feeding off of that. I still have my data here, so I have a couple policies. Let's go have a look at those. I have a policy that's just going to make sure that all my data is matching a JSON schema. And then, clients that are sending bad data, I'm actually just going to drop those clients. So once again, this idea of “we want to make sure the data that is going through our systems is valid, is correct, so that we're not generating insights based on bad data or poor data”. And so, once again, this broker that I might have at the center of my factory, that's a great place for integrations. Within the factory, before we ever get to our hyper scalers, before we ever get to that really expensive compute, we're doing all this still within our factory space.
Magnus McCune: 00:36:48.970 And so, once again, I have the ability to have bridges. I don't have an easy way to demonstrate that from within the Control Center here, but I have a bridge once more that is connecting me across to that enterprise broker, perhaps a Cloud broker, or something that is being run by this customer themselves, and we have a number of clients. Actually, I can show that there's a HiveMQ bridge here that is connected in and sending data. So that's my central broker. One other thing that I want to highlight here is often within these factories, one of the things we'll see — Ravi touched briefly on MQTT Sparkplug, Sparkplug B, and those Sparkplug systems are really important. They're really critical because all of this stuff on the left may speak Sparkplug and you may have a SCADA system that is a Sparkplug-native SCADA system and then is doing a whole bunch of really important information. But as some of you may know, Sparkplug is typically encoded in what's called Protobuf, and that's not really a readable format for an AI system. And so I'll do another quick demo here. I'm running a pre-production version of HiveMQ here, so this isn't a released feature yet. But what we can see is on the left, I have a Sparkplug generator, so this is generating Sparkplug data. This might represent a machine within our SCADA system that is sending out data packets on a specific topic. This is part of the Sparkplug schema — I won't get into it. The problem is, if I have an AI system that's trying to read from this or something like a time series database that is trying to store this, it's not really readable. This isn't useful valid data. It's encoded in Protobuf. And so, HiveMQ has this wonderful feature. Once again, this is built into Data Hub. Let me just expand this slightly. This is built into our Data Hub and allows me to do that normalization or making this data useful right here at the edge. And so, I'll go ahead and enable one of these modules. So we have this HiveMQ Sparkplug to JSON module and I'll go ahead and create an instance of it. We provide some logical defaults as part of this. You can of course modify anything you need here. And I'll go ahead and create that and I will actually not activate upon creation.
HiveMQ Modules and Module Configuration
Magnus McCune: 00:38:55.375 So I'll go ahead and create that and we'll go back to our smaller screen. And so, we can see we're still passing through this sort of encoded serialized data that doesn't make a ton of sense to an AI system. If I was trying to hook up a model to this, it wouldn't make a ton of sense. But if I now go ahead and turn on this policy, we see that instead of this Protobuf serialized data, we're now flowing really nice, contextualized JSON. My AI system can go ahead and start reading this and providing value and insights and sort of really building on top of that. So I just want to show that power of our Data Hub modules and of the Data Hub in general, this idea of “let's get really good data as close to where it's being generated as possible” so that we can really build value on top of that. Just being mindful of time here. So really useful piece of our technology. And then, from there, we're really getting into our sort of core Cloud. So once all of this data within the factory is done, we've generated insights within the factory, we've maybe done some predictive maintenance, we've maybe done some image-based quality inspection. We've sort of done the factory piece. We're now looking maybe at that top floor, that executive who wants to understand performance, who wants to understand how are all of my factories operating, not just this one factory in Munich. And really, this is where that idea of an enterprise Unified Namespace really comes into play. So we may be pushing some of this data into a data lake for long-term analysis and for historical analysis. But what if I want to understand what's happening in my factory right now? And that's really where the idea of this enterprise Unified Namespace comes into play, and I'll show what that looks like. This is going to be a simplified Unified Namespace, but I think it's nonetheless representative of what you might expect.
Understanding What’s Happening in a Factory
Magnus McCune: 00:40:39.603 So I have a — this is our Global Industries Company, and within Global Industries we have a whole number of sites but I'm focusing on just one site today, I'm focusing on Munich. And within our Munich site, we have a number of lines. So we have maybe a storage line and a — or, sorry, a number of areas, I should say. We have a blending area. We have a storage area. We have a number of areas that are within this. And then, within those areas, we may have a number of devices. So in my blending area, I have a mixer. In my storage area, I have a number of silos. In my filling area, I have a number of filling stations, so on and so forth. So this is really that idea of an enterprise Unified Namespace, or, in this case, it's really more focused at the factory site. But you get the idea. We have all of this really great data. And now, we can start building systems on top of this data that really understand not just historically what had happened but at any given moment, what is the current truth? What is that current truth of the systems that I have operating? And this is really where the idea of a Unified Namespace and really where AI systems that can respond in real time come into play. And HiveMQ doesn't build AI systems, that isn't our bread and butter. We're a broker and transportation company. We really are looking to move your data. But as part of that, we understand that our customers want to get a sense of what this might look like. So I have a fun example here, once again, built by my colleague, Anthony, of a UNS bot. So this is an AI presentation. We thought we'd show you some AI. And large language models, I don't think are a core use case in smart manufacturing. But I think they're an easy and approachable way for us to show why having good data is really valuable. And so what we've built today —it's a large language model that is going to query that Unified Namespace. So it's going to get the information from the Unified Namespace and then be able to answer questions.
Asking AI to Extract Information from UNS
Magnus McCune: 00:42:37.666 And this might be something like an executive or someone within your organization who maybe doesn't understand the technical aspects but is looking to get high-level information. So I'll ask some simple questions. I have an option here to go, "What can I ask?" But I'm actually going to skip that. I'm feeling a little sort of brave today. So I'm going to skip that piece. And I'm just going to go and ask my UNS bot a generic question. So what is the current average humidity? We saw earlier that we had some silos. And I just want to understand what is the current average humidity in my silos. So let's go ahead and send the question. This is a live demo. So let's hope everything works. Yeah. So I'm getting a current average humidity. It's saying, "Hey, Silo 1 through 4 have these current humidities. And the average of that is 67.7." So without having to have built a system that is designed to do this, I have a large language model. It's doing some looking up of information for me and then some simple computation for me. And wonderful approach for us it's — once again, HiveMQ doesn't build AI systems. This isn't our goal. But we're trying to demonstrate why having that really valuable knowledge or that really accurate information is valuable. I think that's everything I was looking to cover on the demo side. Ravi, anything you wanted me to touch on that maybe I was quick about?
Ravi Subramanyan: 00:44:02.228 No, I think you touched upon everything. There was actually a question, "Hey, are you going to demo some AI stuff for us?" And my answer is, "Yes." We were going to just wait on and then there you are. You gave an excellent demo. But hey, at the end of the day, we are not an AI company. We are a data enabler, a data management company. So we actually ensure that you have the right data, contextualized, normalized, good quality data is available, and that's what we do, right? So that your AI applications can do what it's doing and not worry about the quality of the data, so.
Magnus McCune: 00:44:32.243 Absolutely. I mean, really, I think that idea of No Data, No AI is really spot on. As I mentioned, I really wanted to add in, No Data is bad, bad data is worse, but certainly the idea of without data you just can't operate. So yeah, that was my demo. Thank you.
Live Q&A
Ravi Subramanyan: 00:44:50.277 Yeah. There are a few questions, Erin. I know a couple of questions seem to be related to cybersecurity, and I think maybe we can just try to address them together. If it's okay, I'll just quickly read it out here. The first one is interesting, "Hey, will this webinar be covering hardware options for secure ways to connect the PLCs, machine tools, and other industrial devices that isolate the device from the network and still allow communication, i.e., CMMC, NIST 800-171 controls." I know this is very specific, but if you want to take a crack at it, Magnus, then I can add my two cents as well.
Magnus McCune: 00:45:24.809 Yeah, absolutely. So I think part of what we're seeing right now is a shift in the cybersecurity model and smart manufacturing. So certainly things like IEC 62443, which is one of the cybersecurity specs for smart manufacturing, is starting to allow for these integrations that are not just those point-to-point integrations we talked about earlier. And one of the really significant parts of that is unlike in a request-response model where sometimes you have to be able to reach down into, say, a level zero, almost all of the — well, sorry, all of the relevant publishing actually happens sort of an outbound direction. And so it actually simplifies security in a number of ways. That doesn't necessarily indicate that it's going to be simple to do this by any means. But I think it allows us to sort of have a more simplified version of security in our smart manufacturing facilities. And so I would say keep an eye on IEC 62443 and have a look at how that changes as we look at these pub/sub-models and specifically this idea of flowing data from the edge without having to have a whole bunch of open ports. The other benefit for me is when we're using MQTT we're sort of guaranteeing, "Hey, we only really have one port that we need to open through our firewalls, through our security devices." We're not having to open http and https and a wide variety of different protocols. We're saying, "Hey, within your level 0, your most secure level, let's have that be an edge device." And then just that one edge device is connecting out of that level 0, into level 1 or 2, or really it ends up being level 3 or 3.5 with our broker, and so really simplifying the number of outbound connections. I hope that answers your question.
Ravi Subramanyan: 00:47:15.742 It does, it does. And there was a follow-up question on, "Hey, are there any — what are your, specifically your cybersecurity offerings that HiveMQ offers?" Maybe briefly touch upon it. I also tried to include our security page that goes into more details, but if you want to quickly touch upon what we do from a security perspective.
Magnus McCune: 00:47:33.918 We're not a cybersecurity company, but of course, our technology is intended to work securely. And so we have really granular role-based access control for our broker. We have our enterprise security extension which can integrate into existing security systems to understand permissions and what systems are accessing what device, and those are the key things — is really securing the flow of that information. Of course, MQTT being a TCP-based protocol, you can do it over TLS. So you can use Transport Layer Security to really encrypt that data in transit. So those are some of the key elements, but fundamentally, we're not a cybersecurity company, but we do want to ensure that the data that we're moving for you is being done so in a secure manner.
Ravi Subramanyan: 00:48:17.102 Yeah. One other thing if I can quickly add, and just because we're not a cybersecurity company, and just because a lot of our customers and prospects came to us and say, "Hey, do you do Active Directory? You do LDAP, you do this, you do that." Right? So what we are saying is that if you're already doing that within your organization, we have something called a Security Extension, which basically acts as a passthrough, where you can basically apply all of the security postures that you already have within your environment on the broker, and make the broker look like it's any other piece of IT software that you have within your ecosystem, and have the same level of security, same level of access controls as you have on your other aspects of your environment, and that is something that is pretty popular. So I just wanted to add that. So good. Let me go to this. Okay. So I think on the demo, basically, does the factory have two sites, or is it only one that was in the diagram? I think it was a representation of maybe subsystems within a factory. But again, I think to me, it's like a factory can be multiple different subsystems. I think with the biggest customers we have, the support is like 70 different factory locations, right? And then within those factory locations, they have, I don't know, 20 plus subsystems that need different ways to collect the information. So it's pretty complicated. But of course, you kind of typically start small. Maybe you start with a factory, you maybe start with a particular subsystem, and then you can work your way from there so that it is not overwhelming from day one. That's kind of what we typically recommend.
Magnus McCune: 00:49:56.201 If I can add to that briefly, the key parts of the idea behind Unified Namespaces, and Unified Namespaces if you're going to use AI, is that idea of a hierarchical data structure that really — that semantic model of the data hierarchy, allows you to, "Hey, we had one factory, now we're adding a second. The rest of the structure remains the same, but we have this sort of hierarchy." And one of the things I really love about that is it makes data really discoverable. If you're looking for the specific value of a silo within a plant in Munich, you start at the root, you start at global industries, and you navigate through until you logically get to the point you're looking for. So it makes the data really discoverable. So while our demo did only contain one site, it could just as easily have contained a dozen. There's no reason it could not, so just more bridge connections.
Ravi Subramanyan: 00:50:47.453 Awesome, awesome. I think there was a question around Sparkplug B subscription. Does it need to be flattened? So if you want to subscribe to a Sparkplug B topic for a specific value, does it need to be flattened, or how do you do that?
Magnus McCune: 00:51:01.577 Yeah, so if you're using — Ravi's diagram showed this nicely which is — if you're using all Sparkplug-enabled systems, then yeah, you're great. You don't have to worry about whether your device's Sparkplug be enabled or not. What I was showing in that module demo is this idea of, "Hey, we actually have the ability to translate from Sparkplug B into JSON." Now as you know, and it seems like you're asking the specific question, which is Sparkplug B has a very specific topic structure. So as part of that same conversion, while I was republishing to the same topic value, I could just as easily publish to a flattened topic structure. So this would be a more complicated transformation, but I could break out, fan out some of that data. I'd have to write that transformation. This is a pre-built one that HiveMQ has built for you, but I can write that transformation and fan out that data.
Ravi Subramanyan: 00:51:57.666 Yeah. And I think our future plan is to make those transformations available and make SDKs available so that you can build your own transformations. Because we understand that you might have some corner cases that we're not already supporting. So you can be — just like how we have the extensions to extend the data from our platform into other applications, and you can create your own custom extensions. You can also, in the future, create your own transformations as well. So there was this other question — yes, please, go ahead. Sorry, sorry.
Magnus McCune: 00:52:26.120 I was going to take the question about the Gemini bot, the one with — [crosstalk]. So whoever accurately guessed that is built with Google Gemini's model, it's the Gemini Pro model. It is entirely reading from the MQTT. So the application interface for that is actually including the MQTT structure, the topic structure, and the payloads in the query across to the Gemini bot so that it has the context of that. There's a really fantastic blog post from my colleague, Anthony, on how that was done. There's actually two blog posts. I think he just published one yesterday or this morning on how to do that with Gemini, and I'll announce it here, which means I really have to do it, but I'm also writing one for how to do that within Azure. So if you're using Gemini, then I would really recommend reading Anthony's blog post, and I'll be looking to write one using the Azure and specifically the OpenAI model, so recommend doing that.
Ravi Subramanyan: 00:53:23.291 Awesome. Awesome, great. So the next question was like, "I'm working with the FactoryTalk Optix, and that's basically like a Rockwell Automation product, and I want to integrate MQTT messaging with software so I can read from Node-RED. Can that be achieved?" I can definitely answer that because I actually was at the Rockwell Automation Conference, and they actually had a demonstration where they were displaying a FactoryTalk Optix, which is basically like a SCADA kind of a system, very similar to ignition if you will. It's the equivalent of that, and they were actually having a demo with Mosquitto in that case, but it can be easily replaced with HiveMQ, talking to Node-RED, talking to Grafana, and being able to show all that information or exchange information, so it is absolutely possible to do that. Great. The next one is interesting. What is the difference — and this goes back to Sparkplug — what's the difference between the Edge of Network (EoN) primary and non-primary application, and Sparkplug? When can the SCADA or brokers be set to primary? Do you want to answer that?
Magnus McCune: 00:54:30.124 I'm going to recommend we follow this one up with some links because Sparkplug begins a fairly complex topic. So I'm going to recommend that we follow this one up with an email or some links because it gets quite into it.
Ravi Subramanyan: 00:54:43.522 Yes.
Magnus McCune: 00:54:44.513 Effectively, your Edge of Network devices are your devices. So whether they're the end device itself or they're masked behind an edge of a network device, they're effectively your devices that are publishing data, your D-data packets, if you will. And then your primary application is the application that is determining whether the whole system is live. And there's some actual downsides to Sparkplug related to primary applications, but I am, by no means, a Sparkplug expert. We have a whole bunch of those at HiveMQ, but I'm not necessarily the one to talk to. So what I'm going to suggest is that we link you to an appropriate article.
Ravi Subramanyan: 00:55:21.354 Yeah. And I shared the Essentials guide. Hopefully, they can start with that and then they can build on top of that. All right.
Magnus McCune: 00:55:27.224 Okay. Yeah.
Ravi Subramanyan: 00:55:28.391 This next one is interesting for you maybe. What is your point of view on using a reverse proxy in a DMZ instead of MQTT bridging?
Magnus McCune: 00:55:35.991 Okay. So certainly, we're using a reverse proxy to create the — so we often use reverse proxies when we're looking to connect across the clusters. That doesn't change the fact that in order to get the data from one broker to another, we still need some way to do so. So even if I have an edge node and a centralized broker and I'm using a reverse proxy to enable that connection, that's fine. But fundamentally, I still need an MQTT bridge through that. Now, if your question is, actually, let's skip the edge broker and just have a broker in the centralized location, let's call that industrial DMZ 3.5. Then yes, absolutely just having a reverse proxy and just having a central broker is totally fine. And a situation in which you might do that is if you don't have a need for protocol conversion, or you're not trying to normalize data right at the edge. So if you didn't have a need to fix or normalize any of that data, and you didn't have a need to convert from a specific protocol, if your system or device spoke MQTT natively, then absolutely just having a broker in the industrial DMZ and having a reverse proxy that enables a connection through to it. Totally reasonable, a worthwhile way to go.
Ravi Subramanyan: 00:56:52.219 Okay.
Magnus McCune: 00:56:53.034 Yeah.
Ravi Subramanyan: 00:56:53.621 Very good, very good. I think we have a couple of other questions. The first one, I can quickly address it, and then I'll ask you the last question. One is — is the DLMS input plugin going to be available in the HiveMQ Edge? And what I understand is DLMS is Device Language Messaging Specification. It is used for communication between smart meters, data concentrators, and utility centers, basically in the energy utilities market. And so we can certainly say that we are kind of like we have built certain of these protocol converters based on key use cases from our customers. We have the common ones there. For example, we built a botnet one for building services. We are continuing to build based on the need, but we also actually provide a very extensive SDK or API system in an environment where customers can actually bring in their own device driver. So if DLMS is something that's important, and you want to look into that with the HiveMQ Edge, we can certainly talk about it. You can develop it yourself, or we can develop it on your behalf as well. So that is something that is open. All right, I think maybe one more quick question. Can you talk about enabling control of processes over MQTT? Some control requirements need redundancy to ensure that control signal can reach and control the intended processes. For example, we may want to enable autonomous control of certain processes from the AI model outputs. So can you kind of address any of these aspects?
Magnus McCune: 00:58:28.446 Yeah, I'm trying to think of how to answer this question. So it is an interesting question. I just am trying to think of the correct way to answer it. [inaudible].
Ravi Subramanyan: 00:58:37.473 That's okay. You know what? Actually, let's maybe table — we'll definitely get back to you with a more detailed response because we are very short on time. Maybe one last question is can we — okay, Unified Namespace, it talks about Unified Namespace and topic structure and hierarchy structure. So how does the hierarchy work in Unified Namespace or the topic structure work in the Unified Namespace? Maybe that's a —
Magnus McCune: 00:58:59.969 Yeah, so with MQTT at the core of your Unified Namespace, then your hierarchy is typically defined through that topic structure. That does, by the way, get a little bit complex with Sparkplug B because Sparkplug B has a very predefined topic structure — we have some great articles on how to handle that. So if you are interested in that specifically, go have a look. There is this idea, though, of how would you create a more dynamic hierarchy for Unified Namespace by bringing together maybe multiple MQTT brokers or multiple topic structures. And that's certainly something we're exploring — what are the different hierarchical models that might exist within a Unified Namespace and how that might look. So what I'd say is keep your eyes on some of the things we're working on and see what might come out of that. Because there are a variety of more dynamic topic structures that are — or sorry, hierarchical structures that are relevant for Unified Namespace concepts. So absolutely something we're thinking about, but MQTT is a rigid topic structure-based hierarchy.
Conclusion
Ravi Subramanyan: 00:59:59.094 All right. So back to you, Erin. I think we are out of time.
Erin Musselman: 01:00:02.907 We are perfectly on time. Thank you so much. Thanks for all the questions today. If we didn't get to you, we will try to get back to you. Otherwise, you can find us at HiveMQ.com/contact to reach us there. Otherwise, thank you to our speakers and everyone for joining. We hope to see you at a future webinar.
Ravi Subramanyan: 01:00:19.992 Thank you.
Magnus McCune: 01:00:20.134 Thanks. Bye-bye now. Okay.
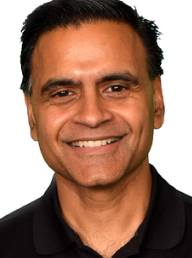
Ravi Subramanyan
Ravi Subramanyan, Director of Industry Solutions, Manufacturing at HiveMQ, has extensive experience delivering high-quality products and services that have generated revenues and cost savings of over $10B for companies such as Motorola, GE, Bosch, and Weir. Ravi has successfully launched products, established branding, and created product advertisements and marketing campaigns for global and regional business teams.
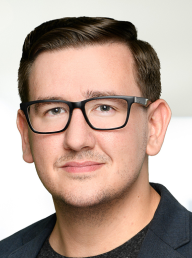
Magnus McCune
Magnus is a Principal Architect at HiveMQ. He is a passionate technologist with a proven background solving complex business and technical challenges through the design, implementation and operationalization of cloud and edge technologies. His expertise extends to network, cloud, & infrastructure architecture, cloud-native solutions design and large-scale automation projects.