Navigating the Snowflake Effect in IIoT Using MQTT
I recently engaged in a conversation with a systems engineer at a global Fortune 500 industrial company, exploring challenges related to standardization and scalability within the context of Industry 4.0. This dialogue led me to delve into a topic I am particularly interested in, referred to as "the snowflake effect."
The snowflake effect points to the unique nature of each site, with the diverse data sources prevalent in large-scale industrial companies including machines, applications, and systems. Typically, these companies operate 50 to 100 sites globally, each specializing in the production of different products. The diversity is a result of a broad product portfolio, decentralized operations, and frequent mergers and acquisitions. While some sites focus on high volume operations, others concentrate on producing a limited number of products, requiring extensive testing to ensure compliance with safety criteria and proper functionality.
Many challenges arise from the distinct nature of each site, featuring various OPC servers, SCADA systems, databases, historians, and a mix of multi-cloud environments, including instances of Azure and AWS. The technological landscape is a mixture of systems and applications implemented at different times, with a significant presence of homegrown systems developed through custom software coding. Addressing this challenge of standardization and scalability is crucial to achieving data-driven decision making and operational improvements across the business.
Standardization and Scalability Challenges in IIoT
Industry 4.0 leaders often focus on standardization, a complicated feat due to the snowflake effect. Concepts such as data modeling, MQTT, MQTT Sparkplug, and Unified Namespace (UNS) have gained traction in the market because MQTT, as a lightweight protocol, offers an ideal method to bring standardization to a complex digital environment. MQTT serves as a universal language that can facilitate a future state of standardization. While understanding the process of assimilating different data types is essential, once translation methods are in place, MQTT can be leveraged to create a common language for all industrial sites.
MQTT Sparkplug is an open-source software specification for MQTT helping the manufacturing industry to seamlessly integrate data from their applications, sensors, devices, and gateways. With Sparkplug, all participants settle on a common data format, how to receive specific data, how to publish their data, and how data can be interpreted.
UNS is a concept that allows the collection of data from various industrial IoT (IIoT) systems, add context to it, and transform it into a format that other systems can understand. It works well with MQTT and Sparkplug.
Creating a Common Reference Architecture Around MQTT and UNS
Let’s consider a fictitious company, Structure Inc. Structure Inc. is a manufacturer of various metal products, including cables and wires, with 60 sites globally. Over the last 30 years, they have acquired four other industrial companies. Many of their sites operate on homegrown software, and they manage a collection of six different SCADA systems, three historians, multiple SaaS applications focused on analytics and machine learning, as well as multiple MES and various other systems. Essentially, it is a highly complex landscape.
To address this complexity, a task force has been assigned to create a standardized approach to data. The team is deeply engaged in understanding concepts like UNS and is fully committed to the idea. The reason MQTT has gained traction, and HiveMQ has become the de facto leader in this space, is because there needs to be a mechanism to establish some form of standardization in this intricate environment. An MQTT broker serves as the bridge, becoming the single source of truth to which other systems can send data.
Ultimately, the key challenge at the forefront of the task force efforts lies in determining the most efficient path to establish a common reference architecture. The reference architecture will act as a template for all plants, providing a standardized framework. While the reference architecture can be adapted and modified to suit unique situations, achieving a common approach is the ultimate goal - a unified method to address the same problem.
In this pursuit, MQTT, Sparkplug and UNS emerge as the optimal solution, serving as the most effective means to navigate and implement the desired standardization across diverse industrial landscapes. What I see when I work with industrial companies, is that when we look to solve the riddle of the snowflake effect, we need a standardized template or methodology we can use as a reference point. UNS is becoming the leading paradigm and MQTT forms the foundation of that template because it facilitates interoperability from various systems, devices and environments in a scalable way.
At HiveMQ we have a wealth of information about how to get started building a Unified Namespace and also on the basics of MQTT. Read up and reach out to me if you would like to discuss how to get started countering the snowflake effect with modern technologies.
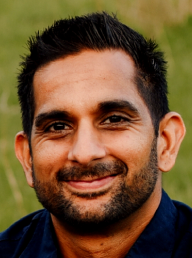
Omar Aziz Ahmed
Omar Aziz Ahmed, Enterprise Account Executive at HiveMQ, leverages over a decade of technology sales expertise to address complex customer challenges. With the last 7 years dedicated to industrial software, focusing on IIoT, Digital Transformation, and Industry 4.0, Omar plays a pivotal role at HiveMQ. His current focus is to help enterprises adopt MQTT to accelerate transformative business outcomes.