Empowering Digital Transformation in Pharma Manufacturing – Pharma 4.0
Pharmaceutical manufacturing faces several pressures that are impacting their ability to bring safe and effective medicines from R&D to market faster and overcoming issues like supply chain disruptions and competition. This needs to be done in a cost-effective manner without compromising on quality and efficiency. In addition, the pharmaceutical world is highly regulated and compliance management is both time-consuming and costly, while non-compliance can lead to significant fines or legal action.
Introducing Pharma 4.0
The pharmaceutical world is increasingly turning to the integration of digital technologies and automation to solve these problems. This approach has been termed Pharma 4.0, which is a concept that is borrowed from Industry 4.0. Basically, Pharma 4.0 involves the use of advanced technologies such as artificial intelligence, machine learning, robotics, and Industrial IoT to improve efficiency, quality, and safety in drug research and development, manufacturing, and distribution. The goal of this initiative is to:
Improve efficiency: Automate the entire process across manufacturing lines and locations.
Enhance quality control: Apply real-time monitoring and analytics to quickly identify and fix issues before they become endemic in the process.
Innovate faster: Use digital technologies to respond to change faster, and quickly identify and recover from costly mistakes or unsuccessful endeavors.
By doing this, the hope is that high-quality cost-effective medicine can be brought to the market faster so that pharma companies can focus more on the research of new drugs.
Data and Pharma 4.0
Collecting, understanding, and analyzing data has become an essential component of Pharma drug manufacturing and is key to adopting Pharma 4.0. Pharma companies generate vast amounts of data daily, from lab experiments to manufacturing to supply chain management. This data has the potential to help Pharma manufacturers make better-informed decisions, optimize processes, and drive growth in the following areas:
Compliance
The pharmaceutical industry is probably the most highly regulated industry, and compliance with regulations such as Food and Drug Administration (FDA), Medicines & Healthcare products Regulatory Agency (MHRA), European Medicines Agency (EMA), and others can be extremely challenging. Ensuring compliance requires accurate data tracking, zero data loss, secure storage, and reporting, which is challenging without data automation.
An effective compliance program is essential to prevent business disruptions and loss of reputation from failed audits. As a result, Pharma manufacturers must maintain strict quality control, detailed product information, digital batch records, and continuous data integration between operational technology (OT) and information technology (IT) systems.
Operational Efficiency
Operational efficiency refers to the enablement of high visibility, informational transparency, robust security, effective work management and process tracking in Pharma manufacturing. As there are a number of factors that need to be taken into consideration, it has become more and more challenging for Pharma manufacturing companies to bring operational efficiency.
One way to achieve this is by applying sophisticated algorithms on the process data available which has been possible with better computational power and storage capabilities. Instead of intuition, the new normal is to rely on data to drive digital innovations and operational efficiency decisions. Indeed, data is the “most valuable resource” for Pharma manufacturing organizations.
Quality Control
The aim of quality control in Pharma manufacturing is to verify and test the medicine at various stages of production, to ensure every product is of the highest quality. Quality control also involves identifying any defects in products and fixing these problems with corrective techniques and measures.
Pharma 4.0 – The Journey
As shown in Figure 1, the journey from data collection to digital maturity in Pharma manufacturing is one in which analysis, context, and insights are added to transform raw data captured from a device or system into information, knowledge, and, finally, actionable wisdom for decision makers.Figure 1: The stages of the data maturity model on the path to realizing Pharma 4.0.
First, data is collected from Pharma manufacturing machines/processes like feeding machines, wet granulation machines, flatbed dryers, compressors, lyophilizers, and similar equipment. They are then normalized, digitized, and organized as Big Data. Next, meaning (or labelling) is added, and data is synthesized into knowledge via AI. Finally, the data is transformed into actionable wisdom attained through the combined insights of digital maturity.
Data Collection - The First Frontier
The first and most significant frontier to achieve digital maturity for Pharma 4.0 is data collection and movement. Data from Pharma manufacturing machines, processes, and applications are captured and stored via key ingestion technologies. On the operational technology (OT) side, data is stored with controllers, PLCs, gateways, and edge devices, and on the IT side, it is stored in a data center or enterprise cloud. Data storage technology enables the long-term storage of digitized data captured from advanced sensors and systems. This data-rich environment enables advanced initiatives such as machine learning, AI, adaptive control, and digital twins.
Pharma Data Collection Challenges
There are some challenges to data collection and data movement in Pharma manufacturing. Machines and processes in the Pharma manufacturing plant are heterogeneous and use various protocols to communicate. Data generated during Pharma manufacturing can vary significantly in format, quality, and completeness. This can make collecting, integrating, and analyzing data difficult.
Data connectivity is also a major issue due to factory systems’ archaic, legacy nature. As a result, typically, IT and OT systems don’t have an easy way to communicate to enable Pharma 4.0 initiatives.
Industrial IoT (IIoT), a subset of Pharma 4.0, uses smart sensors and actuators along with software to consolidate the data to enhance manufacturing and industrial processes. IIoT is a key enabler in achieving OT IT convergence and the interoperability of the various communication protocols. It creates a data abstraction layer in the middle and a common data language to translate the various communication protocols to enable interoperability.
Figure 2: IIoT serves as the intersection point between OT and IT systems
This OT IT convergence matters because success in today’s connected industrial landscape hinges on collaboration. IIoT is changing how manufacturers work, blurring the lines between IT and operations. For example, IT pros might now spend more time working with equipment on the factory floor, while OT teams must focus on cybersecurity and networking best practices. IT-OT convergence isn’t about turning IT pros into heavy machinery operators or plant engineers into data scientists. Instead, it’s about creating a strategy that bridges the gap and allows organizations to improve operational performance by working around a unified set of objectives and KPIs.
Pharma Data Collection Solution - MQTT and the Data Broker
A Data Broker is a key technology enabler for IIoT to enable this data abstraction layer. A data broker is an intermediary entity that enables OT and IT client systems to communicate with each other. Using an underlying standard such as MQTT, a data broker supports the ability to connect multiple clients publishing data and multiple clients that are subscribed to receive the data such as enterprise applications. The clients communicating with the broker can abstract the underlying protocol that the machines/processes use to communicate. The broker works well in low bandwidth environments with unreliable communication mechanisms due to the underlying publish/subscribe method, where machines/processes don’t need to keep polling to get the data.
MQTT is a standard binary publish-subscribe messaging protocol designed for fast and reliable data transport between devices, especially under very constrained conditions. Constraints include unreliable network connectivity, limited bandwidth, limited battery power, and similar limited conditions. It is built on top of TCP/IP, which is the go-to communication protocol to interconnect network devices on the Internet. MQTT is ideal for IIoT due to the reasons mentioned above.Figure 3: How an MQTT messaging system works
The MQTT data broker can securely communicate the data between publishing clients, typically on the OT side, to subscribing clients on the IT side. For example, an MES application might want data from the SCADA system to run its analytics to identify batch variability easily. The MES application would run an MQTT client subscribed to the broker. The SCADA client would publish data to the broker when available. As a result, the MES application subscribed to the broker would automatically get the updates without needing to poll for the data.
MQTT technology is designed to push data to and from thousands of remote devices across numerous sites to the enterprise. Sparkplug is a framework that sits on top of MQTT to add more context to the Manufacturing data. It is an open-source software specification that provides MQTT clients with a framework to integrate data and provide context by defining data models. It provides a consistent way for pharma manufacturing equipment manufacturers and software providers to share contextual data, accelerating the digital transformation of existing operations.
Sparkplug allows IIoT deployments to decouple the data between hardware and software sources. With Sparkplug, new data sources are immediately discoverable to other system components, and these sources can become a single source of truth. Sparkplug is fully secured, requiring no open ports for new devices and requiring TLS for all data transport.
Pharma Data Collection Solution - Architectural Overview
Figure 4 below provides an MQTT Sparkplug-based data architecture which shows how the data broker connects multiple machines/processes and applications to enable seamless bidirectional data movement.
This architecture solves the pharma manufacturing challenges of data integration and real-time data movement while providing efficient and secure data transfer to support digital transformation initiatives.Figure 4: MQTT Sparkplug-based data architecture that supports multiple Pharma manufacturing data producers and data consumers to bridge OT to IT
Figure 5 below shows how data collection and movement are managed in a pharma-manufacturing plant and external systems to enable a global supply chain optimization use case. Pharma supply chain managers want to trigger necessary workflows from a centralized location based on leading indicators derived from sourcing, supplier inventory, and shipping. Supply chain performance data can flow through an MQTT Broker to the cloud provider’s infrastructure, where it can be ingested and then analyzed or monitored. The MQTT data broker serves as the single source of truth for all of the pharma manufacturing supply chain data coming from various sources to enable supply chain managers to track all the KPIs on their supply chain and detect any anomalies they can react to.Figure 5: Shows a way to enable Pharma supply chain optimization using a data broker that enables proactive tracking of key supply chain KPIs and detect anomalies
Next Steps: Explore HiveMQ’s Solution for Pharma 4.0
As discussed in this article, the move to Pharma 4.0 is real and a business-critical initiative. Data is the most valuable in Pharma manufacturing, and this forms the basis and yardstick for improving efficiency, quality, and safety in drug development, manufacturing, and distribution. We have helped many Pharma companies embark on their journey to Pharma 4.0 and welcome the opportunity to discuss a solution tailored to your business needs.
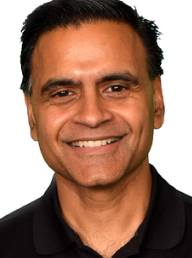
Ravi Subramanyan
Ravi Subramanyan, Director of Industry Solutions, Manufacturing at HiveMQ, has extensive experience delivering high-quality products and services that have generated revenues and cost savings of over $10B for companies such as Motorola, GE, Bosch, and Weir. Ravi has successfully launched products, established branding, and created product advertisements and marketing campaigns for global and regional business teams.